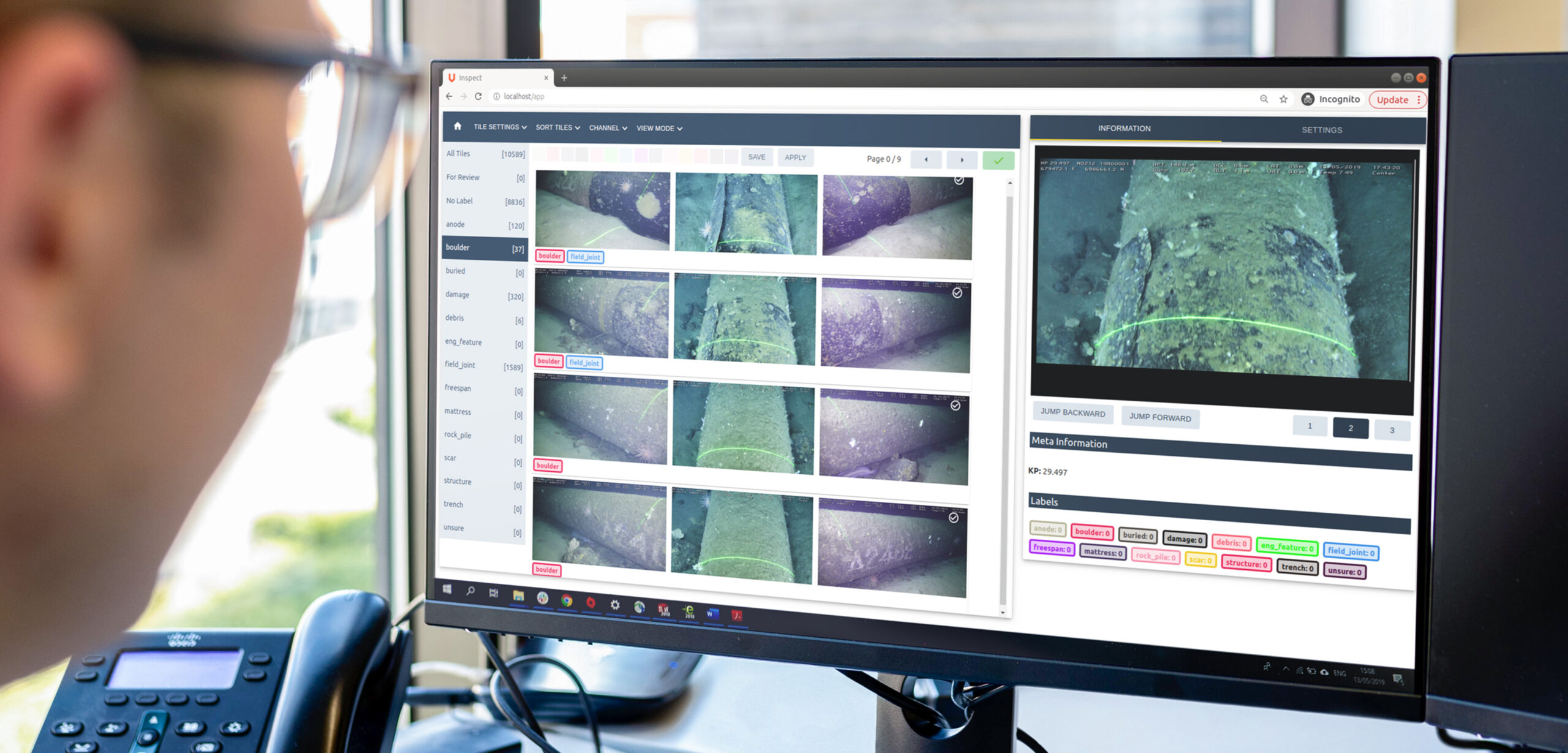
Vaarst’s ML Platform for pipeline inspection
A human in the loop approach to machine learning:
Vaarst’s Machine Learning (ML) Platform is a solution to quickly event and QC hours of survey datasets in a fraction of the time and with a higher quality output. The unique “human in the loop” approach of our solution gives the human operator a powerful tool that will take away the tediousness of repetitive tasks and focus their effort where it matters, by critically examining significant images that the system has automatically selected for revision.
Vaarst was offered a dataset from a survey of a Shell-operated 120Km pipeline undertaken in 2019 by iSURVEY. This dataset was analysed using our ML Platform software, with the objective of assessing its performance and speed gains when compared to using traditional methods.
The events categories used in the exercise were the following: field joints, anodes, debris, anomalies and rockpiles. Two extra categories were added as a stretch target to identify freespan and burial.
The exercise was carried out in two phases:
Phase 1 – Eventing using ML Platform’s semi-automatic tools
Using our Semi-Automatic tools the user is able to speed up data eventing by grouping similar images together and identifying images that contain unique visual features. In accordance with the specific requirements of each survey, the user can manually add event labels to images and use ML Platform to intelligently propagate such labels to similar images. This is the key aspect of our approach, as it is far more flexible when compared to other attempts structured around a black-box, fully automated ML approach, which are typically only capable of eventing a pre-defined fixed set of classes.
There are three main ways of grouping images:
- Similarity search (single image): user selects a target image and the system will rank all other images by similarity to the target image.
- Similarity group search: images are ranked by similarity to a specified group of images selected by the user. In essence, an ad-hocML model is trained on the fly.
- Outlier search:ranks the images such that those appearing to be visually distant from the rest of the data are ranked highly. This feature is useful for identifying anomalies and solves one of the crucial limitations of the black-box attempts mentioned above.
In this phase of the project, the system did not use any supervised ML models and it did not exploit any prior information about the asset to be inspected or the events of interest.
Phase 2 – Eventing using ML Platform’s machine learning models
In this phase we built ML models to automate the eventing, using approximately 1/3 of the data generated in Phase 1. We demonstrated how an operator using the ML Platform can accurately event a dataset even more quickly when ML is used to assist them. This eventing speed gain is due to the fact that in our proposal the operator has to review only those portions of the data for which the ML models do not provide a confident detection.
Using Vaarst’s ML Platform software, the 120Km dataset could be evented in only 6 hours 20 minutes when all the ML features and module are used. When freespan detection is included, the dataset could be evented in 16 hours and 20 minutes. It is important to note that this is based on freespan detection using visual data only, further speed gains can be obtained when data from other sensors such as Multibeam is taken into consideration by the operator.
High quality, consistent results in a fraction of the time
Results obtained with the Shell-iSURVEY Pipeline Dataset show that the ML Platform tools give users the ability to reduce eventing time while offering high quality and consistent results. The operator has access to two levels of assistance: semi-automatic tools and ML models.
Semi-automatic tools are based on a “human in the loop” framework that offers a significant reduction of eventing time with respect to traditional methods.
Using this approach, freespan eventing for 10Km of data was completed on average in 2.5 hours, while all remaining events type were annotated on average in 4 hours per 10km of data.
Eventing time can be further reduced with the usage of pre-built ML models created using as little as 1/3 of the data generated using semi-automatic tools. The operator will then further refine the output by only intervening in instances highlighted by the system for review, where Machine Learning alone could not provide a reliable result.
The operator also has the ability to adjust the confidence thresholds of the ML model and set it to an optimal level depending on the events category, thus avoiding instances of false positive and false negatives.
The estimated time for object detection eventing decreased to 6 hours and 20 minutes from the original 48 hours required to complete the full dataset using semi-automatic tools.
The estimated time for free-span eventing decreased to 10 hours from the original 30 hours required to complete the full dataset using semi-automatic tools.
Improved, collaborative workflows
The main advantages of ML Platform are reduced eventing time and high-quality eventing. In addition, there are several other aspects of this product that make it a game-changing tool for subsea pipeline inspection.
The software presents a common platform that can be used by different stakeholders at different stages of operations to achieve correct interpretation, thus extending the lifetime and usability of results.
The survey contractor provides the first level of review, which the Operator/QC contractor can quickly access and review in more detail. Both survey contractor and Operator/QC contractor can work closely and more efficiently on the same dataset, further increasing the level of detail of the report at each step, saving time and reducing chances of missed events.
Once the eventing and reporting are complete, the Operator can access the evented dataset in the future as well, conducting specific searches using ML-based similarity and anomaly searches. The deliverables from the survey contractor are now interactive and easy to access, generating insights that can be used in planning future surveys and ad-hoc maintenance campaigns.
Cost savings are generated not only by reduction of personnel and time, but also by faster planning and increased knowledge of the conditions of the assets.
Similar stories
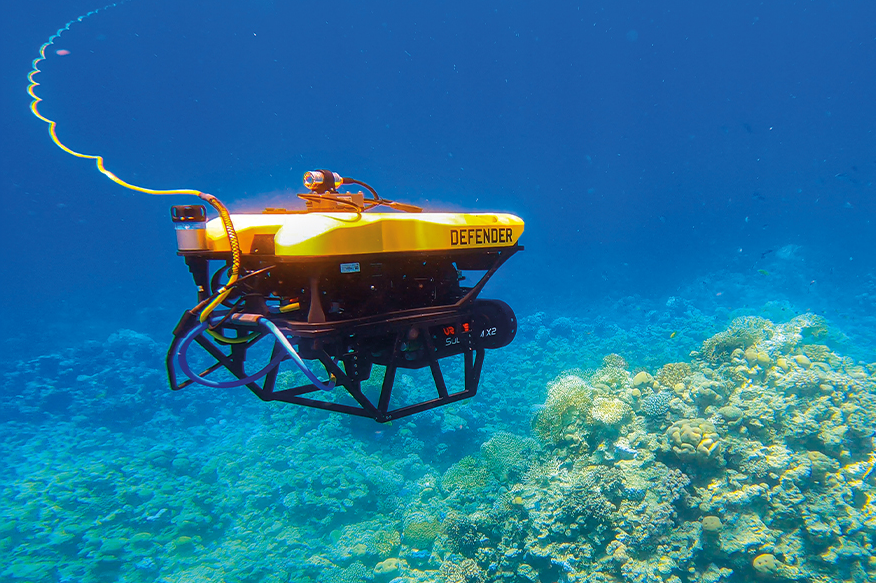
We are proud to announce our collaboration with Red Sea Global (RSG), a visionary developer spearheading regenerative tourism projects worldwide.…